The $30 AI Breakthrough: Berkeley Researchers Challenge the Cost of Innovation
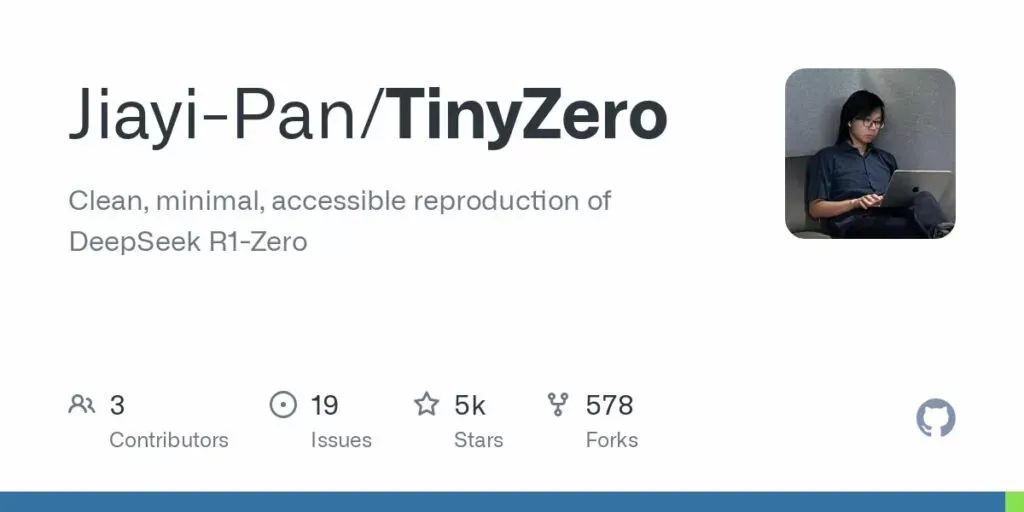
A Game-Changing Discovery in AI Research
A team of researchers from the University of California, Berkeley, has sent shockwaves through the artificial intelligence community by claiming they have replicated the core technology behind DeepSeek’s groundbreaking AI—all for a mere $30.
This revelation adds a new twist to the ongoing debate over whether cutting-edge AI development necessitates billion-dollar budgets or if alternative, cost-effective approaches have been overlooked by major tech firms.
DeepSeek’s Disruptive Entry into AI
DeepSeek recently gained widespread attention by unveiling R1, an AI model that purports to rival the capabilities of ChatGPT and other expensive machine-learning systems, but at a fraction of the typical training cost. The announcement turned heads in Silicon Valley, as many assumed such high-performance AI models required massive computational power, energy consumption, and vast financial investment.
However, the Berkeley team sought to push the limits of affordability even further. Led by PhD candidate Jiayi Pan, the researchers developed a smaller-scale AI model, dubbed TinyZero, which they have made publicly available on GitHub for experimentation. While TinyZero does not match the 671-billion-parameter scale of DeepSeek’s R1, Pan asserts that it successfully mimics the fundamental behaviors seen in DeepSeek’s “R1-Zero” model.
Reinforcement Learning on a Budget
The key to TinyZero’s functionality lies in reinforcement learning, a technique where the AI, beginning with random guesses, gradually improves its responses by refining and searching through potential solutions. In a blog post detailing the project, Pan highlighted the Countdown game—a British television puzzle that requires players to combine numbers to reach a target value—as a prime example of their AI's learning process.
"The results: it just works!" Pan wrote. Initially, the AI produced nonsensical answers, but over time, it adapted and learned from its mistakes. This simple demonstration underscores the effectiveness of reinforcement learning, even on an ultra-low budget.
A Challenge to the Conventional AI Model
The idea that a sophisticated AI function can be replicated with just a few days of work and $30 is a wake-up call for the tech industry. Traditionally, AI breakthroughs have been associated with massive data centers, high-powered GPUs, and multi-million-dollar investments. DeepSeek had already challenged this notion by claiming to develop its model at a fraction of what U.S.-based firms like OpenAI and Google typically spend. Now, Pan’s work suggests the price could be even lower.
Yet, not everyone is convinced. Skeptics warn that DeepSeek’s claims of affordability may not provide the full picture, as the company might be leveraging undisclosed resources or proprietary model distillation techniques. Additionally, while TinyZero demonstrates that reinforcement learning can be executed on a shoestring budget, it remains unclear whether it can perform the vast range of tasks that larger AI systems handle. Rather than a direct competitor, TinyZero may be more of a proof-of-concept demonstrating how reinforcement learning can be effectively scaled down.
Support The Journal
SupportThe Future of AI: Leaner, Smarter, Cheaper?
The implications of TinyZero’s success go far beyond a single experiment. If researchers and open-source developers can replicate high-end AI capabilities with minimal resources, it raises significant questions about why industry giants continue to invest billions in AI infrastructure. Is the high cost of AI development truly necessary, or have inefficiencies and inflated costs been built into the current AI ecosystem?
Certainly, large-scale AI models require extensive computational power to support advanced capabilities. However, the emergence of leaner AI alternatives suggests that at least some aspects of AI development could be streamlined. Open-source projects like TinyZero may pose a real challenge to established players by demonstrating that AI can be more accessible and affordable than previously assumed.
A New Era of AI Accessibility?
For Jiayi Pan and his team, the goal is clear: “We hope this project helps to demystify the emerging RL scaling research.” Their findings have already ignited a broader discussion about AI accessibility and efficiency. If innovations like TinyZero continue to emerge, the AI landscape could shift from a domain controlled by a handful of tech giants to one where smaller teams and independent researchers play a far greater role.
Whether this breakthrough will fundamentally reshape AI development or remain a niche experiment, one thing is certain—the conversation around affordable, powerful AI is only just beginning.